Summary
Sports analytics is the analysis of sports data, including features such as player performance, related business operations, recruitment, scouting, etc. Sports analytics utilizes the application of mathematical and statistical rules to sports.
In this article we will give you a comprehensive introduction to sports analytics
What is Sports Analytics?
Various data-capturing technologies have emerged available in the sports business in recent years. These new technologies allow sports management to capture and collect data on games, bidding, bookmaker odds, playing styles, scores, and many other sports features.
There are now a number of different ways that analytics can be used in sports. Some teams use analytics to help with player recruitment and contract negotiations. Others use analytics to track player movements on the field and to improve their performance.
Data analytics in sport has become an integral part of sport business
Different types of Sports Analytics
Generally speaking, sports analytics is typically divided into on-field and off-field analytics.
On-field Sports Data Analytics
Coaches and managers have been using analytics to track player performance and make better decisions on the field. This means that you focus on player tracking, which uses data to improve movement and positioning on the field.
There is a lot of work that goes into on-field analytics in order to optimize player fitness and performance. This involves the use of metrics to track things like how fast players are running, how far they are sprinting, and how many jumps they are making. By using this data, teams can better understand how to improve their players’ performance.
There is a growing trend in the use of on-field analytics to optimize performance, such as player fitness. By tracking player metrics during games, teams can make better decisions about how to improve their players’ performance. This can have a significant impact on the team’s success.
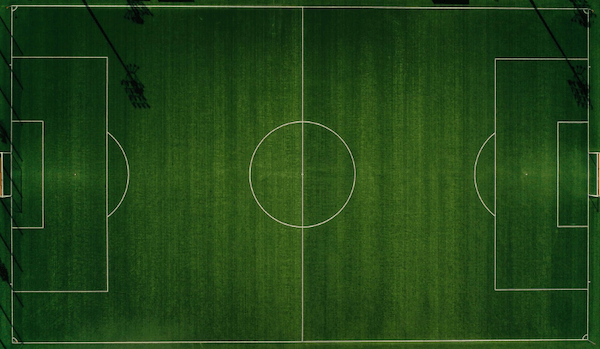
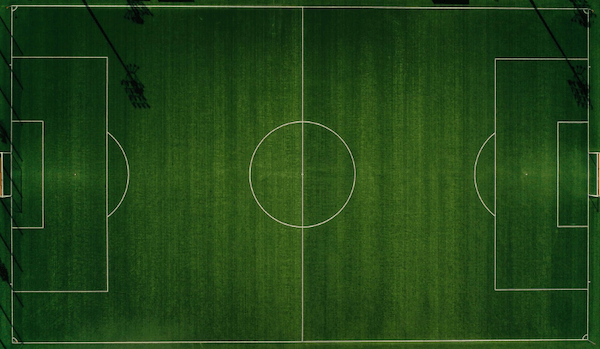
Off-field Sports Data Analytics
Off-field analytics deals with the business side of sports analytics. In other words, off-field sports analytics is the process of analyzing data that is not directly related to the game itself. This data can come from a variety of sources, including social media, ticket sales, financial records, and so on.
Off-field analytics encompasses everything in the sports business outside of on-field performance. It focuses more on business aspects such as sales and ticket pricing. This data is used to help sports organizations grow their business and increase profitability.
For example, off-field analytics involves the use of data to surface patterns and insights that can help improve ticket and merchandise sales, as well as fan engagement.
Questions examined could for example be:
- What actions should we take to increase game attendance?
- Which sales channels will be most effective for us?
- How will the global sporting landscape affect our business?
- Which group of fans should we target with which products and how?
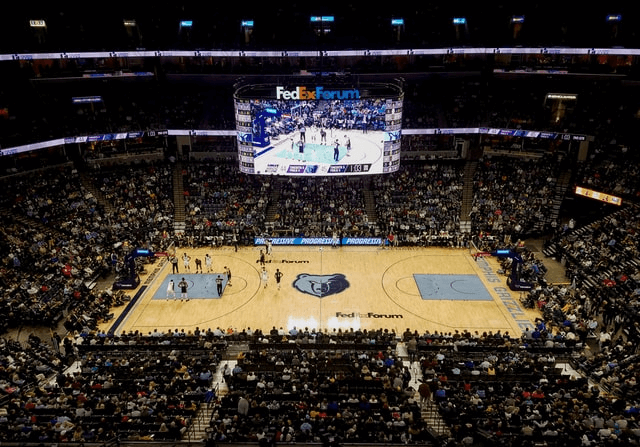
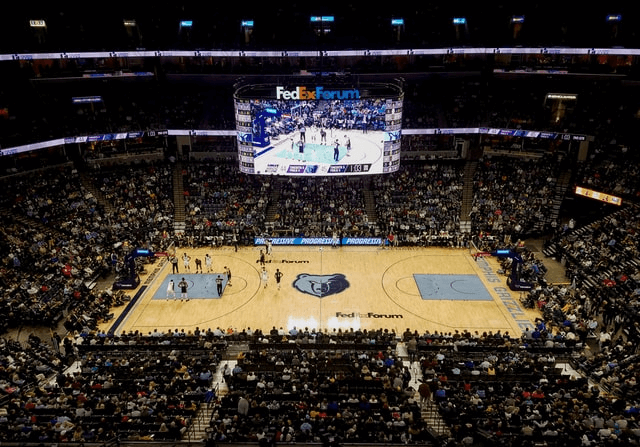
How is Sports Analytics used in Sports? Use Cases for Sports Data Analytics
Sports analytics are the application of data analytics in the field of sports. It has been used in a number of sports, including football, cricket, baseball and basketball.
There is no doubt that sports analytics has become an important part of the sports world. The use of analytics in sports has been growing for a while now and it is used in a variety of ways.
One way that analytics is used in sports is to improve player performance. This can be done by tracking things such as how much time a player spends on the court, how many shots they take, and where those shots are taken from. This data can then be used to help players improve their games.
In basketball, for example, analytics can be used to measure player movements on the court, shot efficiency, and which players are most effective in specific areas of the court. This data can then be used to help coaches make better decisions about who to play and how to play them.
Another way is to assess team performance, such as how well they are performing compared to other teams. Sports analytics can also be used to predict the outcome of games or matches.
Examples of Sport Analytics: Examples of How Technology Is Changing The Game
There are many examples of how sport analytics is changing the game. Let’s have a closer look at two of them
Example 1. How Miami Heat uses Microsoft Power BI to transform their customer interactions and business operations
This is one of my favorite examples and one I refer to often, how the NBA team the Miami Heat used the analysis and visualization features to transform its customer interactions and business operations completely.
By using business intelligence across almost the entire organization, with an adoption of 70% across the organization, the Miami Heat increased season ticket sales by 30% and saves approximately $1 million on operations.
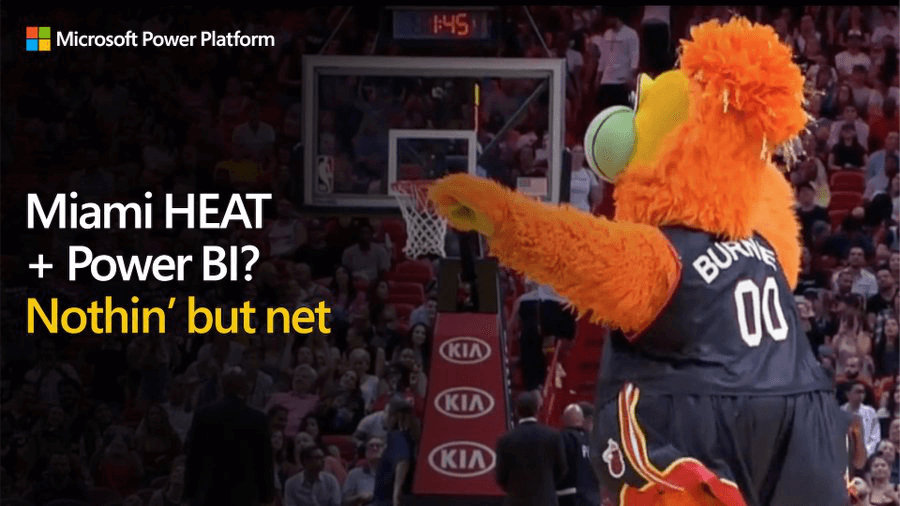
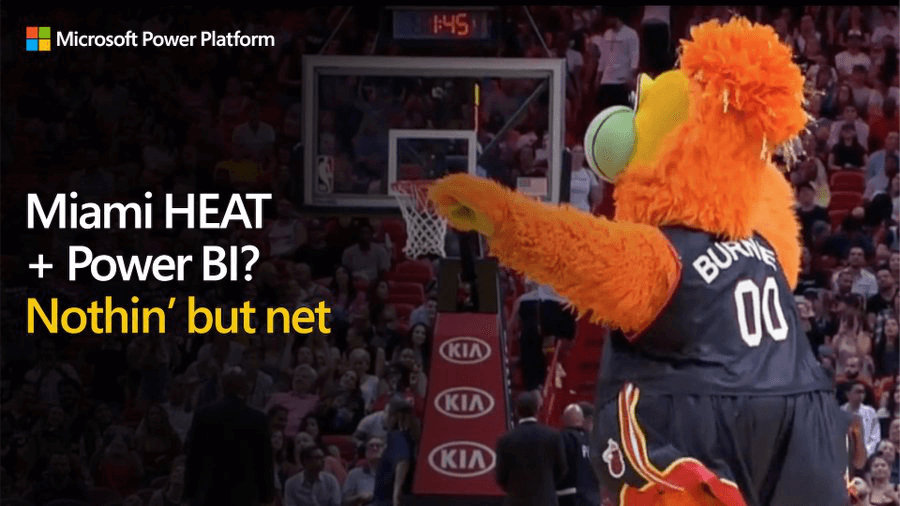
Image source: Microsoft Power BI
How they did it? The main thing was that they got a much better understanding of the different customer needs and how they could fulfill and exceed these needs.
If you want to see the full example I recommend watching the video on the Microsoft website in the link: Microsoft Docs Power BI
Example 2. Real Madrid Partner with Microsoft
Real Madrid F.C., one of the greatest football clubs in the world, has joined forces with Microsoft to use technology to transform its operations, performance, fitness, and relationships. Their collaboration is ranging from on-field analytics to off-field analytics.
Real Madrid F.C. has been working with Microsoft to transform their organization using data and digital technology that streamlines operations, enables coaches to tailor performance and fitness methods, and gives supporters unparalleled access to their favorite team.
Some examples of the sport analytics Real Madrid are using:
- Predicting and preventing injury
- Evaluating for optimal performance
- Maximizing training utilizing data
- Tracking fan behavior
- And much more …
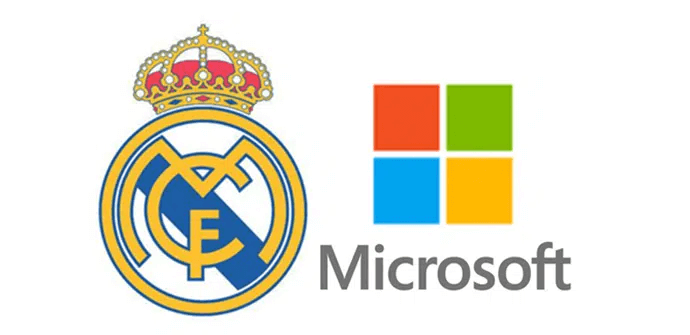
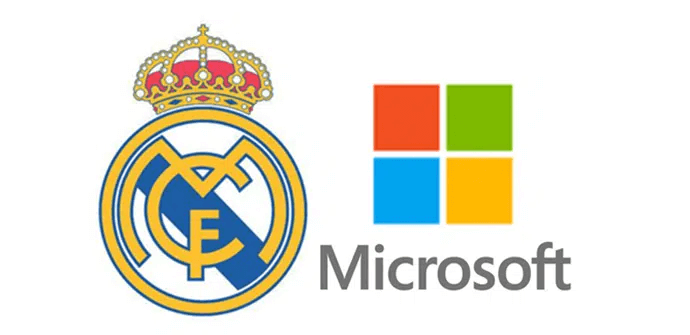
How to Get Into Sports Analytics? 4 Steps to Get Started as a Sports Data Analyst
If you’re interested in a career in sports analytics, there are a few steps you should consider to take in order to get started.
1. Get the Necessary Skills
The first step is to acquire the necessary skills. This includes learning how to use statistics and analytics software, as well as understanding business concepts. Get experience in mathematics, statistics, programming, and data analysis.
Having strong analytical skills involves being able to collect and analyze data in order to identify trends and insights. You must also be able to communicate complex data analysis in a way that is easy for non-experts in data analytics to understand.
2. Gain Experience
This can be done by working as an intern or volunteer for a sports team or organization. Sports analysts require experience to perform well in their roles. Experience gives you an advantage when attending job interviews and also shows that you have interest in the field.
Many major sports organizations now have departments dedicated to analytics. This includes professional sports teams, collegiate athletic departments, and even international organizations like FIFA. There are also many private companies that provide sports analytics services.
3. Choose an Area of Specialization
There are numerous areas of specialization that you could choose to focus on. Picking just one can be a difficult task. To give you some examples on specializations in sports analytics if you are looking to get into the field
- Player analysis: This would involve studying player movement, tendencies, and performance in order to help teams make better personnel decisions
- Tactical analysis: This would focus on studying game footage in order to identify trends and weaknesses in teams’ offensive and defensive strategies
- Business analytics: This area would involve analyzing off-field activities and focuses more on business aspects such as, finances, sales, pricing, and so on. Additionally, analytics can also be used to understand the fan base and how to appeal to current and new fans
- Scouting and Talent Identification: There are many different aspects of scouting and talent identification that can be analyzed with analytics. Some of the most common areas include: player movements, physical attributes, performance data, and financials. By analyzing all of this data, scouts and coaches are able to get a better understanding of potential signings
No matter which specialization you choose, it is important to have a strong foundation in the basics of sports analytics. Once you have a strong foundation, you can begin to focus on the specific area of specialization that interests you the most.
4. Establish Contacts In The Industry
A key aspect when starting in sports analytics is to build a strong network of contacts in the industry. This will help you get your foot in the door and start building relationships with key people.
One way to build your network is by attending sporting events and meeting people in the industry. You can also attend conferences and networking events specifically for sports analytics professionals. In addition, you can reach out to people online who work in sports analytics and connect with them on social media.
Sports Data Analyst Career and Salary Opportunities
According to Glassdoor, the estimated total pay for a Sports Data Analyst is $73,486 per year in the United States area, with an average salary of $62,963 per year.
Similarly, comparably estimates that the average Sports Analyst in the US makes $78,356. Furthermore, the salaries of Sports Analysts in the US range from $14,286 to $383,751 , with a median salary of $69,424 according to comparably.
7 Most Used Tools for Sports Data Analysis
There are several tools that are being used in sports analytics available on the market, below are some of the most used
Python
Python has become the most popular programming language in the world for a reason – it is versatile and easy to learn. For sports analytics, Python is the perfect tool. It can be used to track athlete performance, analyze game data, and predict outcomes.
Python has already been used by several top sports organizations, including the NBA, MLB, and Manchester City FC. Python allows analysts to work with large data sets and make quick and accurate predictions. Additionally, Python makes it easy to create visualizations of data, which can help teams and organizations better understand their performance and make better decisions.
If you are interested in sports analytics, Python is the language for you.
R
R is a programming language and software environment for statistical computing and graphics. R programming is used extensively in sports analytics. It helps analysts make better predictions about the performance of players and teams.
Additionally, it can be used to improve the understanding of how games are played, and to identify potential weaknesses in teams and players.
R programming is also used to develop models that can be used to analyze player and team data. This information can then be used to improve decision-making by coaches and managers.
Power BI and Tableau
Power BI and Tableau are business analytics tool used to make data-driven decisions. It can be used to track and analyze player and team performance in sports.
One way Power BI and Tableau can be used in sports analytics is to track player performance. This includes tracking things such as how many goals a player scores, how many assists they make, and how many tackles or blocks they make. Power BI and Tableau can also be used to track player injury data. This can help teams decide when to rest players, and help them come up with strategies for avoiding injuries.
One example of how Power BI is used in sports analytics is the Golden State Warriors. The Warriors use the software to track player movement on the court, as well as shot attempts and makes. This information helps them to decide which players to put in the game, and where to position them on the court.
Excel
Excel is used extensively in sports analytics. It is used to track player and team stats, develop strategies, and predict outcomes. In many ways, Excel has become the unofficial tool of sports analytics.
One of the main ways Excel is used in sports analytics is to track player stats. This can be done manually, or more often, it is done using Excel’s built-in formulas and functions. Excel can also be used to create graphs and charts of player stats, which can then be used to help develop strategies.
SQL
Sports teams collect data, a ton of it. In order to make this data readily accessible, they usually store it in large structured databases
SQL is a database query language that is used to query and analyze data. It can be used to track player and team statistics, as well as to evaluate player and team performance.
SQL allows people to query data, filter data, and group data in order to better understand sports performance. It is also used to create reports and track player and team stats.
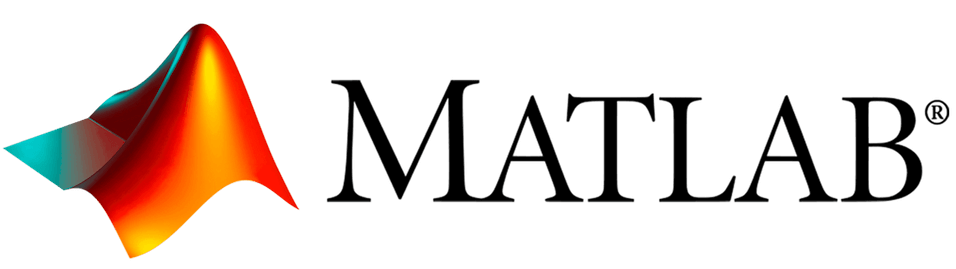
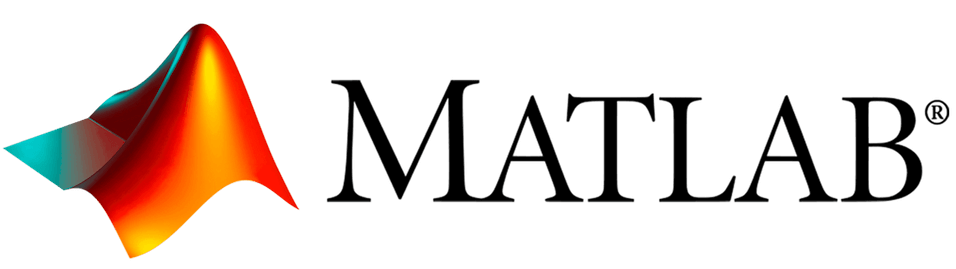
Matlab
Matlab is used to develop algorithms and models to analyze sports data.
One common use is to develop models of player or team behavior. These models can then be used to predict the outcome of future games or races. Matlab can also be used to optimize training regimens for athletes, or to evaluate new strategies during a game.
Apache Spark
Apache Spark is used for sports analytics to process the increasing amount of data that is generated during sports games. This data is used to improve player and team performance. Spark can handle large amounts of data quickly, which is necessary for sports analytics.
It can also analyze data in real-time, so that coaches and players can make decisions during the game. Apache Spark is used in sports analytics to improve the accuracy of player and team predictions.
Challenges in Sports Analytics
There are a variety of challenges that come with sports analytics
01
Sports are Unpredictable
One of the biggest challenges is that sports are unpredictable. Even with all of the data in the world, it’s impossible to know what is going to happen on the field. This unpredictability makes it difficult to create reliable models and algorithms.
One of the biggest challenges in sports analytics is accounting for human variability. Athletes and teams are not machines; they often make unexpected decisions or perform differently than expected.
02
Data Quality
The quality of sports data can vary, and it is important to ensure that the data is accurate and reliable before using it for analysis. Inadequate or inaccurate data can lead to flawed analytics and decision-making.
Some common sources of data quality issues in sports include:
- Incomplete or incorrect data. For example, if an athlete’s stats are not accurately tracked, it can lead to inaccurate conclusions about their performance.
- Biased data. Data collected from certain sources may be biased due to factors such as personal opinion or financial interest.
03
Developing Accurate Models
Models help analysts understand the game, identify key trends, and predict future outcomes. Inaccurate models can lead to faulty analysis and bad decision-making.
One of the biggest challenges in developing accurate models is accounting for human variability. Athletes and teams are not machines; they often make unexpected decisions or perform differently than expected. Additionally, weather conditions, referee decisions, and luck can all impact game outcomes.
All of these factors make it difficult to develop accurate models in sports analytics. However, with careful data collection and analysis, it is possible to create models that give a more accurate representation of reality.
04
Communicating the Findings at Appropriate Level
Communicating the findings of sports analytics to decision makers can be difficult as it requires a level of understanding about complex statistical models. The findings of sports analytics are often complicated and difficult to communicate.
One way to overcome this challenge is to focus on storytelling. Analysts can use storytelling to explain how their findings impact the game and how they can improve performance. They can also use storytelling to show how analytics can be used to benefit fans, players, and the club.
FAQ: Sports Analytics Introduction for Beginners
What is Sports Analytics?
Sports analytics is the process of gathering, analyzing and interpreting data in order to improve performance in sports. This can include using statistics to evaluate players, predicting outcomes of games, and improving coaching methods.
Sports analytics has become increasingly important in recent years as teams have sought to gain a competitive edge. Organizations have employed specialists in sports analytics to analyze data and help make better decisions. The use of analytics has led to improvements in a variety of areas, including player recruitment, team strategy, and stadium design.
What are some examples of Sports Analytics?
Some common examples of sports analytics include tracking player movement and position on the field, analyzing game footage to look for trends and weaknesses, monitoring player health and injury prevention, and studying opponent strategies.
How much do sports analyst make?
According to Glassdoor, the estimated total pay for a Sports Data Analyst is $73,486 per year in the United States area, with an average salary of $62,963 per year.
Similarly, comparably estimates that the average Sports Analyst in the US makes $78,356. Furthermore, the salaries of Sports Analysts in the US range from $14,286 to $383,751 , with a median salary of $69,424 according to comparably.
How do I become a Sports Data Analyst?
There is no one specific path to becoming a sports data analyst. However, there are some commonalities among the best analysts in the field.
The most important trait for a sports data analyst is an understanding of statistics and analytics. Most successful analysts have degrees in mathematics, economics, or computer science. However, some have backgrounds in sports management or journalism.
An analytical mind and a passion for sports are essential for success in this field. It is also important to be up-to-date on the latest trends and developments in the sports world.