Key takeaways
- Big data and business intelligence go hand in hand as big data provides the raw data for BI tools to analyze and extract insights.
- Big data contributes to the generation of predictive and prescriptive analytics, enabling organizations to anticipate future trends and make proactive decisions. Business intelligence platforms facilitate the dissemination of these insights to support decision-making processes.
- Big data encompasses vast and varied data sources, including structured, unstructured, and semi-structured data. Business intelligence leverages these data sources to extract meaningful insights and drive strategic actions.
Big data is a term that refers to large and complex data sets that traditional data processing tools and techniques cannot manage.
In this post, we’ll look closer at big data in business intelligance and how it’s reshaping the way companies gain insights, make strategic moves, and stay ahead of the competition. Big data and business intelligence go hand in hand as big data provides the raw data for BI tools to analyze and extract insights.
Understanding Big Data
When it comes to business intelligence, one term that you’ll hear a lot is “big data.” Big data refers to large and complex data sets that are difficult to process and analyze using traditional data processing tools.
Defining Big Data
Big data is a term used to describe data sets that are so large and complex that they cannot be processed by traditional data processing tools. These data sets can come from a variety of sources, including social media, mobile devices, and internet-connected sensors.
The sheer size and complexity of big data make it difficult to analyze using traditional data processing tools, which is why new technologies and techniques have been developed to help manage and analyze it.
Characteristics: 5 V’s of Big Data
There are three main characteristics that define big data: volume, variety, and velocity.
1. Volume: Amount of Data
Big data is characterized by its sheer volume. Traditional data processing tools are simply not equipped to handle the massive amounts of data generated by modern businesses. As a result, new technologies have been developed to help manage and analyze these large data sets.
2. Variety: Diversity of Data
Big data comes in many different forms, including structured, semi-structured, and unstructured data. Structured data is data that is organized and easily searchable, such as data stored in a database.
Semi-structured data is data that is partially organized, such as data stored in a spreadsheet. Unstructured data is data that has no predefined structure, such as social media posts or customer reviews.
3. Velocity: Speed of Data Generation
Big data is also characterized by its velocity, or the speed at which data is generated and processed. Modern businesses generate data at an unprecedented rate, which means that traditional data processing tools are simply not fast enough to keep up.
New technologies have been developed to help process and analyze data in real-time.
4. Veracity: Accuracy of Data
Veracity emphasizes the quality and reliability of the data. It underscores the need for accurate, trustworthy data to derive meaningful insights and make informed decisions.
5. Value: Worth of Data
The ultimate goal of big data is to extract value from the information it holds. By analyzing and interpreting big data, organizations can uncover valuable insights that drive innovation, efficiency, and strategic decision-making.
Unstructured vs Structured Data
One of the key differences between structured and unstructured data is that structured data is organized and easily searchable, while unstructured data has no predefined structure.
Structured data is typically stored in a database, while unstructured data can come from a variety of sources, including social media, mobile devices, and internet-connected sensors.
The challenge with unstructured data is that it is difficult to analyze using traditional data processing tools. However, new technologies such as artificial intelligence and machine learning have been developed to help manage and analyze unstructured data.


Business Intelligence Basics
If you’re new to the world of business intelligence, it can be helpful to start with the basics. In this section, we’ll cover the evolution of business intelligence, BI tools and platforms, and the role of BI in decision-making.
Evolution of Business Intelligence
Business intelligence has come a long way since its origins in the 1960s. In the early days, BI was focused on simple data analysis, but today’s tools and platforms are much more sophisticated. With the rise of big data, BI has become an essential tool for businesses of all sizes.
BI Tools and Platforms
There are many different BI tools and platforms available, each with its own strengths and weaknesses. Some of the most popular options include Tableau, Microsoft Power BI, and QlikView. These tools allow businesses to collect, analyze, and visualize data in a way that is easy to understand.
Example of a dashboard in Tableau
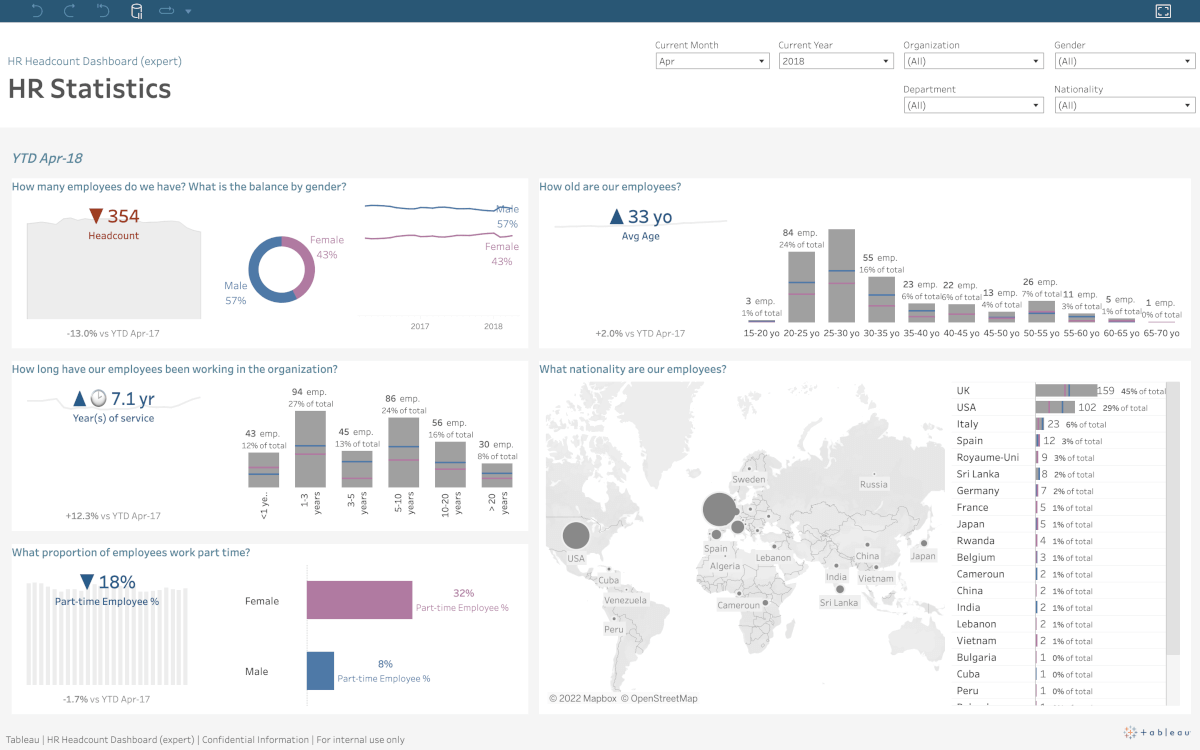
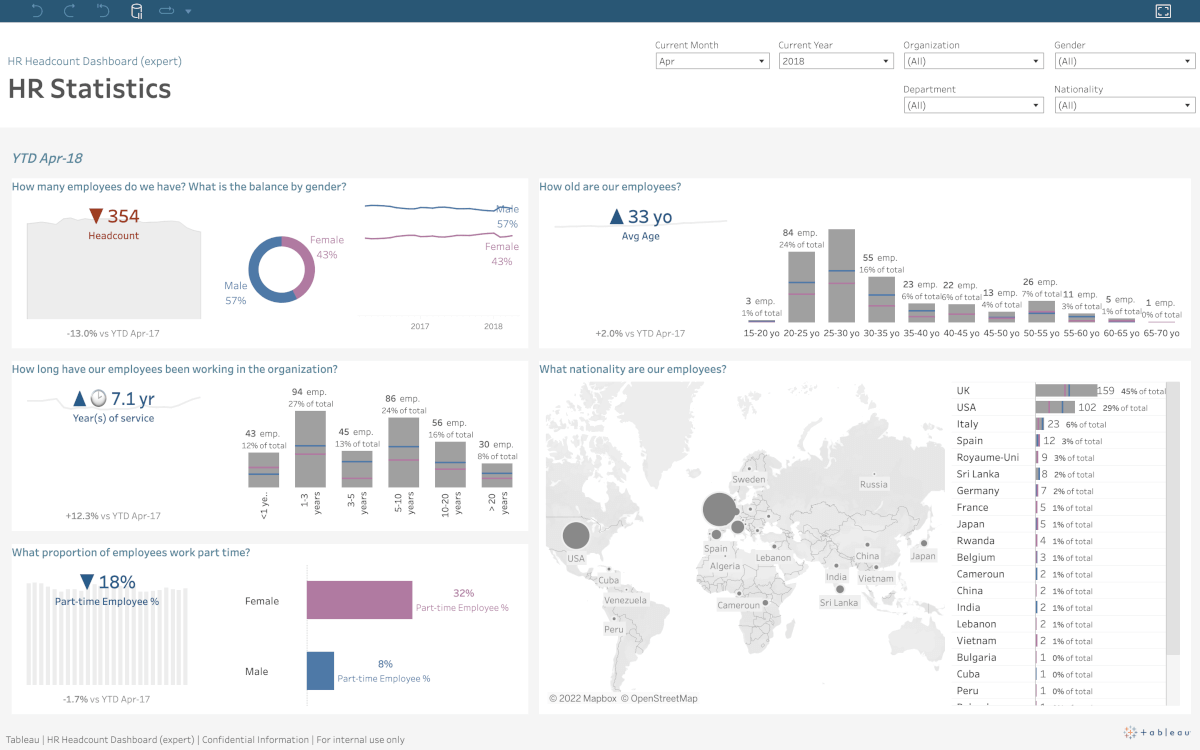
Role of BI in Decision-Making
One of the primary benefits of business intelligence is its ability to provide insights that can inform business decisions. By analyzing data from various sources, BI tools can help businesses identify trends, patterns, and opportunities that might otherwise go unnoticed. This can lead to more informed decision-making and ultimately better business outcomes.
Big Data Analytics with Business Intelligence
Big data analytics has become an integral part of business intelligence. It involves analyzing large and complex datasets to extract valuable insights that can be used to make data-driven decisions. In this section, we will discuss some of the analytics techniques used in big data analytics.
Analytics Techniques
There are various analytics techniques used in big data analytics. They include descriptive analytics, diagnostic analytics, predictive analytics, and prescriptive analytics.
- Descriptive analytics involves analyzing historical data to gain insights into past events.
- Diagnostic analytics involves identifying the reasons behind past events.
- Predictive analytics involves using statistical models and machine learning algorithms to predict future events.
- Prescriptive analytics involves recommending actions based on the insights gained from predictive analytics.
Predictive and Prescriptive Analytics
Predictive and prescriptive analytics are particularly useful in big data analytics. Predictive analytics helps businesses to identify patterns and trends in their data, which can be used to forecast future events.
Prescriptive analytics, on the other hand, helps businesses to make decisions based on those predictions. For example, prescriptive analytics can be used to determine the best course of action to take in response to a predicted event.
Data Mining and Machine Learning
Data mining and machine learning are also important techniques used in big data analytics. Data mining involves extracting knowledge from large datasets.
Machine learning involves building models that can learn from data and make predictions based on that learning. These techniques are particularly useful in identifying patterns and trends in data that may not be immediately apparent.
Process Mining
Process mining is another technique used in big data analytics. It involves analyzing event logs to gain insights into business processes. Process mining can be used to identify bottlenecks and inefficiencies in business processes, which can then be addressed to improve efficiency and productivity.


Importance of Big Data in Business Intelligence
Big data plays a pivotal role in shaping the landscape of modern business intelligence, providing organizations with valuable insights and strategic advantages. Here are some key areas:
- Informed Decision-Making: Big data empowers organizations to make data-driven decisions by analyzing large and diverse data sets, leading to more informed and strategic business choices.
- Uncovering Market Trends: Through advanced analytics, big data enables businesses to identify emerging market trends, consumer preferences, and industry patterns, allowing for proactive adaptation and innovation.
- Enhanced Operational Efficiency: By leveraging big data analytics, businesses can optimize operational processes, improve resource utilization, and streamline workflows, leading to increased efficiency and productivity.
- Personalized Customer Experiences: Big data enables the creation of personalized customer experiences by analyzing customer behavior, preferences, and feedback, leading to enhanced customer satisfaction and loyalty.
- Competitive Advantage: Businesses can gain a competitive edge by harnessing big data to identify new revenue streams, market opportunities, and areas for growth, enabling them to stay ahead in a dynamic business environment.
Relationship between Big Data and Business Intelligence
Big data and business intelligence are closely related concepts, with big data serving as the raw material that is used to fuel business intelligence initiatives. Business intelligence involves the collection, analysis, and presentation of data in a way that is meaningful and actionable to business decision-makers.
Here’s a breakdown of the relationship between big data and business intelligence:
- Data Sources: Big data encompasses vast and varied data sources, including structured, unstructured, and semi-structured data. Business intelligence leverages these data sources to extract meaningful insights and drive strategic actions.
- Data Processing: Big data technologies enable the storage, processing, and analysis of massive datasets. Business intelligence platforms utilize these processed datasets to generate reports, dashboards, and visualizations for decision-making purposes.
- Analytics Capabilities: Big data analytics tools uncover patterns, trends, and correlations within large datasets. Business intelligence tools utilize these analytics capabilities to provide actionable insights to stakeholders at all levels of the organization.
- Decision Support: Big data contributes to the generation of predictive and prescriptive analytics, enabling organizations to anticipate future trends and make proactive decisions. Business intelligence platforms facilitate the dissemination of these insights to support decision-making processes.
- Strategic Planning: Big data supports strategic planning by providing a comprehensive view of market dynamics, customer behavior, and competitive landscapes. Business intelligence platforms aid in translating this information into actionable strategies and initiatives.


Data Management and Tools to Handle Big Data For Business Intelligence
When it comes to managing and analyzing big data, having the right tools is essential. In this section, we will cover some of the most important data management and analysis tools that you should be aware of.
Databases and Data Warehouses
Databases and data warehouses are essential tools for managing large volumes of data. A database is a collection of data that is organized in a way that allows for easy access, retrieval, and management. A data warehouse is a type of database that is specifically designed for handling large volumes of data.
Some of the most popular databases and data warehouses include Oracle, SQL Server, and AWS Redshift. These tools allow you to store and manage large volumes of data, and provide a range of features for querying and analyzing that data.
Hadoop and Spark Ecosystems
Hadoop and Spark are two of the most popular big data processing frameworks. Hadoop is an open-source software framework that allows for the distributed processing of large data sets across clusters of computers.
Some of the components of what is called the Apache Hadoop ecosystem


Spark is another open-source framework that is designed for large-scale data processing.
Both Hadoop and Spark are used for a wide range of big data applications, from data processing and analysis to machine learning and artificial intelligence. These frameworks are highly scalable and can handle large volumes of data with ease.
Self-Service BI and Visualization Tools
Self-service BI and visualization tools are designed to make it easy for non-technical users to analyze and visualize data. These tools allow you to create interactive dashboards and reports, and provide a range of features for exploring and analyzing data.
Some of the most popular self-service BI and visualization tools include Tableau and Power BI. These tools allow you to create stunning visualizations and reports, and provide a range of features for exploring and analyzing data.
Example of a dashboard in Power BI


Big Data and Business Intelligence Jobs
If you’re interested in a career in Big Data and Business Intelligence, there are several roles and responsibilities that you can pursue. In this section, we will discuss the different roles in Big Data, Business Intelligence, and overlapping responsibilities.
Roles in Big Data
Big Data is a rapidly growing field, and there are several roles that you can pursue in this area. Some of these roles include:
- Big Data Engineer: As a Big Data Engineer, you’ll be responsible for designing, building, and maintaining the infrastructure required to process and analyze large volumes of data. You’ll also be responsible for implementing data security and privacy measures.
- Data Scientist: Data Scientists are responsible for analyzing large volumes of data to identify patterns and trends. They use statistical analysis and machine learning algorithms to develop predictive models and make data-driven decisions.
- Data Analyst: Data Analysts are responsible for collecting, analyzing, and interpreting large volumes of data. They use data visualization tools to present their findings and make recommendations to stakeholders.
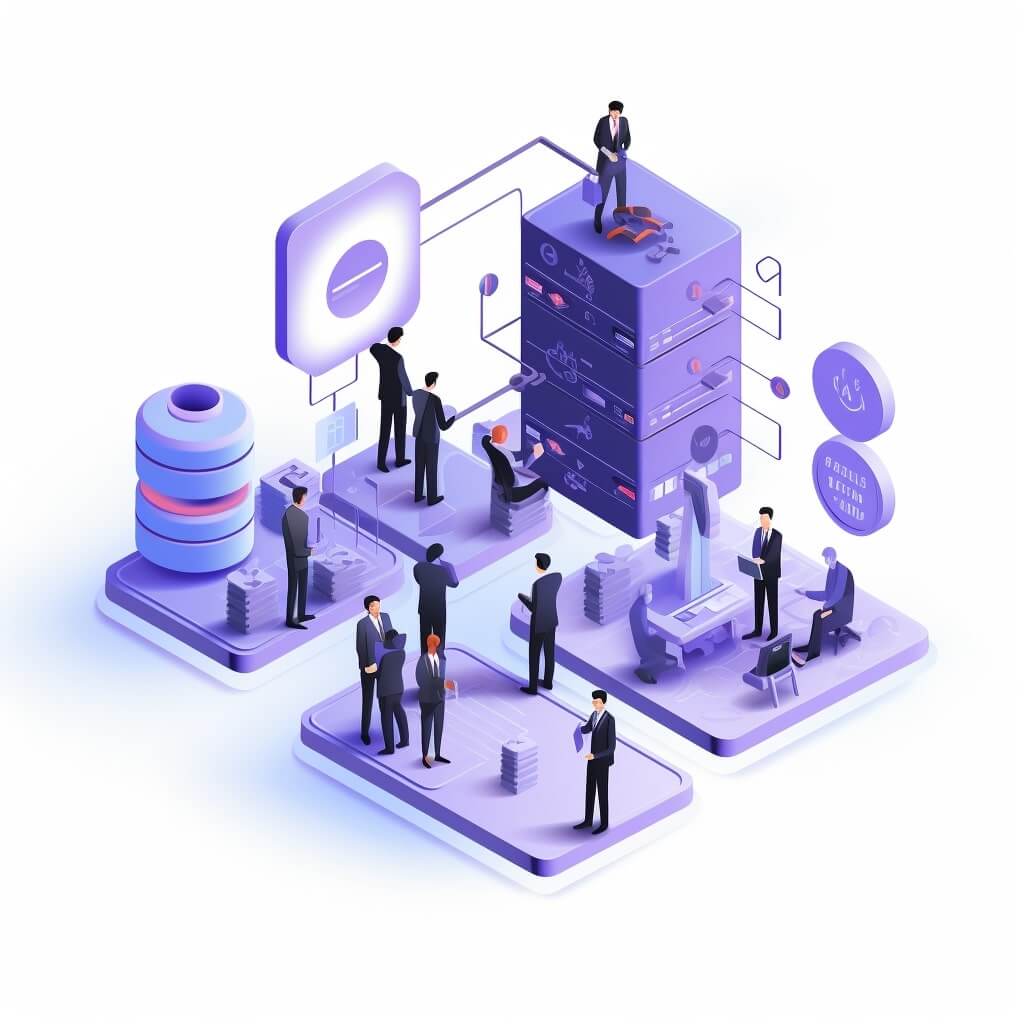
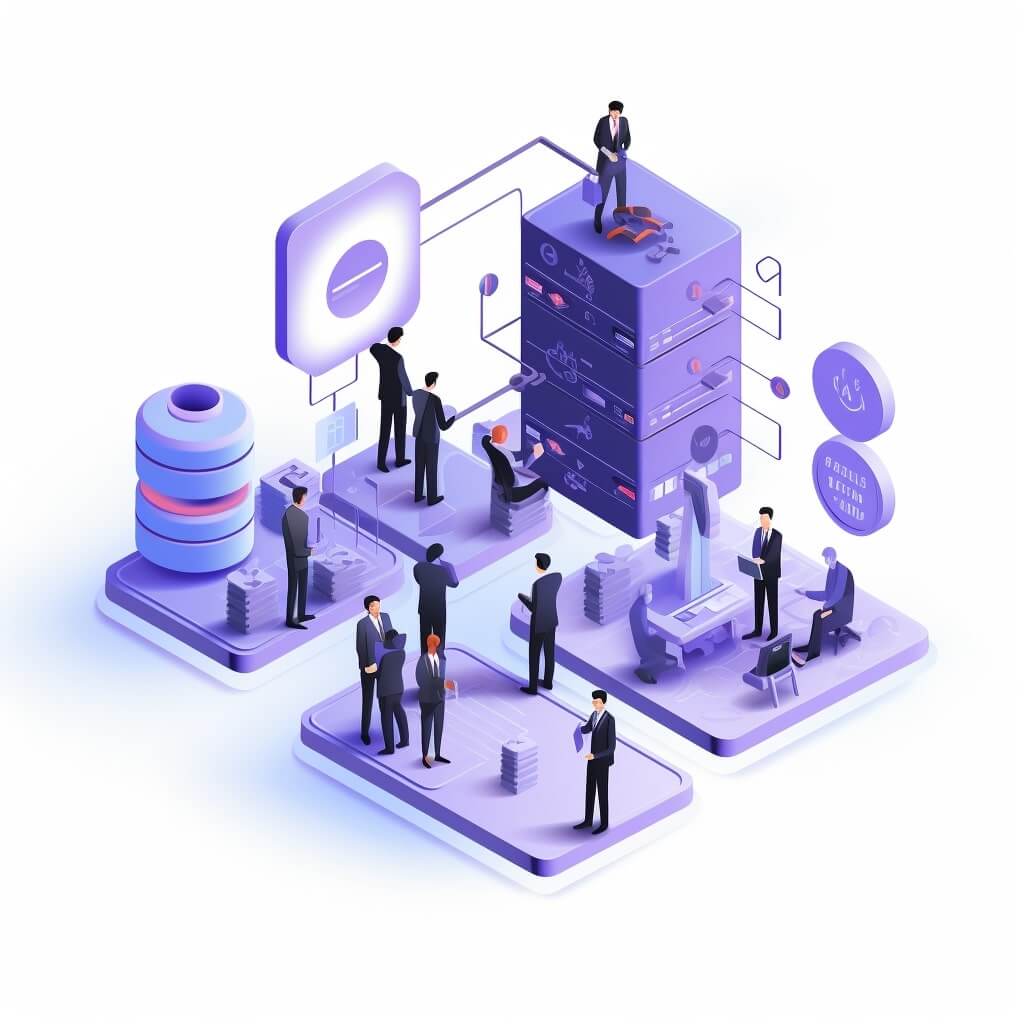
Roles in Business Intelligence
Business Intelligence is a field that focuses on using data to drive business decisions. Some of the roles in Business Intelligence include:
- Business Intelligence Analyst: Business Intelligence Analysts are responsible for collecting, analyzing, and interpreting data to help businesses make informed decisions. They use data visualization tools to present their findings and make recommendations to stakeholders.
- Business Intelligence Developer: Business Intelligence Developers are responsible for designing and developing data models and reports. They work closely with Business Intelligence Analysts to ensure that data is accurate and up-to-date.


Overlapping Roles and Responsibilities
There are several roles in Big Data and Business Intelligence that share overlapping responsibilities. For example:
- Data Architect: Data Architects are responsible for designing and maintaining the data architecture of an organization. They work closely with Big Data Engineers and Business Intelligence Developers to ensure that data is stored and processed efficiently.
- Data Engineer: Data Engineers are responsible for building and maintaining the infrastructure required to process and analyze large volumes of data. They work closely with Data Scientists and Business Intelligence Analysts to ensure that data is accurate and up-to-date.


In conclusion, there are several roles and responsibilities that you can pursue in Big Data and Business Intelligence. Whether you’re interested in data analysis, data engineering, or business intelligence, there are plenty of opportunities available.
Future Trends in Big Data and BI
As we move towards the future, Big Data and Business Intelligence (BI) are becoming increasingly intertwined. Here are some of the trends that we can expect to see in the coming years.
Artificial Intelligence and IoT
Artificial Intelligence (AI) and the Internet of Things (IoT) are two of the most exciting trends in technology today. As more and more devices become connected to the internet, the amount of data that is being generated is increasing exponentially.
AI can help us make sense of this data by analyzing it in real-time and providing us with insights that we can act on.
Advanced Analytics and Cloud Computing
Advanced analytics and cloud computing are two trends that are already having a significant impact on the world of Big Data and BI.
With cloud storage becoming more affordable and accessible, businesses are now able to store and process vast amounts of data without having to invest in expensive hardware. This has made it possible for smaller businesses to take advantage of the benefits of Big Data and BI.
Real-time Processing and Edge Computing
Real-time processing and edge computing are two trends that are set to revolutionize the way we think about Big Data and BI.
Real-time processing allows us to analyze data as it is being generated, providing us with insights that we can act on immediately. Edge computing, on the other hand, allows us to process data closer to the source, reducing the amount of time it takes to analyze and act on it.
In the digital economy, businesses that are able to make sense of their data and act on it quickly are the ones that will succeed. By staying on top of these trends, you can ensure that your business is well-positioned to take advantage of the benefits of Big Data and BI.


Tips: If you are curios to learn more about business intelligence and related topics, then check out all of our posts related to business intelligence
Business Intelligence with Big Data: The Essentials
The intersection of big data and business intelligence presents a wealth of opportunities for organizations seeking to harness the power of data-driven insights.
As companies navigate the complex landscape of information, big data continues to play a pivotal role in shaping the future of business intelligence, enabling informed decision-making and strategic advancements.
Key Takeaways: Big Data and Business Intelligence
- Data Integration: Big data integration is essential for comprehensive business intelligence, consolidating diverse data sources for holistic insights.
- Predictive Analytics: Leveraging big data for predictive analytics empowers organizations to forecast trends and make proactive decisions.
- Scalability and Flexibility: Big data technologies offer scalability and flexibility, accommodating the growing volume and variety of data for robust business intelligence.
- Real-Time Insights: Big data facilitates real-time analysis, allowing businesses to derive immediate insights and respond swiftly to dynamic market conditions.
- Competitive Advantage: Effectively leveraging big data within business intelligence strategies provides a competitive edge, driving innovation and growth.
FAQ: Business Intelligence and Big Data
How does big data analytics enhance decision-making in businesses?
Big data analytics provides businesses with valuable insights that can be used to make informed decisions. By analyzing large volumes of data from various sources, businesses can identify patterns, trends, and relationships that may not be immediately apparent. This can help businesses make more accurate predictions about customer behavior, market trends, and other factors that can impact their bottom line.
What are some common applications of big data in various industries?
Big data is being used across a wide range of industries, including healthcare, finance, retail, and manufacturing. In healthcare, big data is being used to improve patient outcomes by analyzing large amounts of patient data to identify trends and patterns. In finance, big data is being used to identify fraudulent transactions and to improve risk management. In retail, big data is being used to personalize marketing messages and to optimize product offerings. In manufacturing, big data is being used to improve production efficiency and to reduce waste.
Can you provide examples of how companies have successfully used big data?
One example of a company that has successfully used big data is Amazon. By analyzing customer data, Amazon is able to make personalized product recommendations to each customer, which has resulted in increased sales and customer loyalty. Another example is Netflix, which uses big data to make personalized movie and TV show recommendations to its customers.
What are the key features that distinguish big data analytics from traditional data analysis?
The key features that distinguish big data analytics from traditional data analysis are volume, velocity, and variety. Big data analytics deals with large volumes of data that are generated at high velocities and are of different varieties. Traditional data analysis, on the other hand, deals with smaller volumes of structured data that are generated at slower velocities.
How has the integration of big data transformed business intelligence practices?
The integration of big data has transformed business intelligence practices by providing businesses with more accurate and timely insights. With big data analytics, businesses can analyze large amounts of data in real-time, which allows them to make more informed decisions. This has led to improved business performance and increased competitiveness.